We are teaming up with the Simulation Center at UT Southwestern to build the future of medical eduction.
-
Advanced AI tools to automatically analyze, grade, and provide immediate feedback to medical students and other health professionals in training.
-
Multi-modal Foundation Models for text, video, and audio to comprehensively perceive medical student encounters with patient actors
-
Improving the quality, consistency, and efficiency of medical education training
Automatic Zero-Shot Grading of Medical Student Notes with Large Language Models ("Rubric to Prompts")
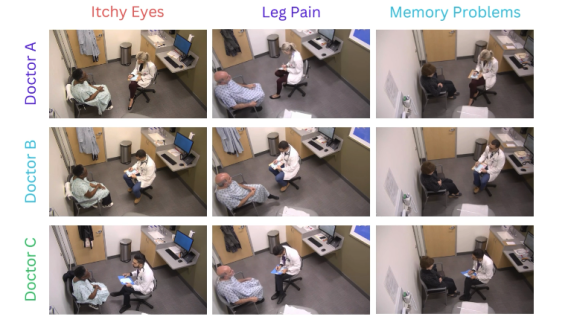
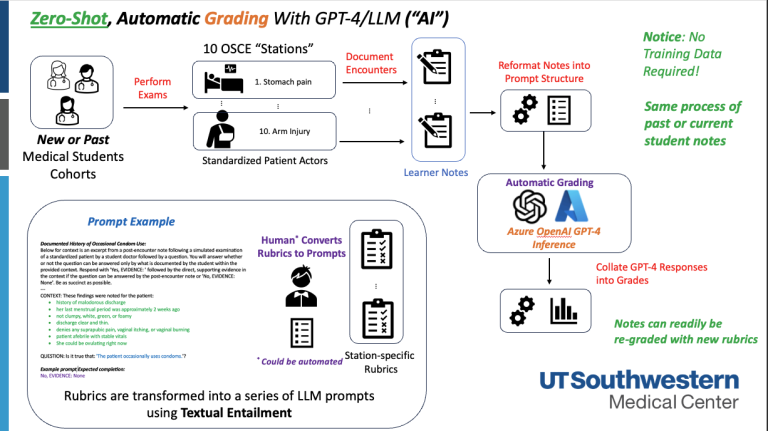
Not just grades, but also expanding to tailored Narrative Feedback for medical students and educator mentors.
Rubrics to Prompts: Expert-level Performance Assessment and Feedback in Medical Education with Foundation Models The project aims to establish and evaluate the effectiveness of AI-generated feedback on medical students’ post-encounter notes. Leveraging a dataset of 15,000 existing examples, the project plans to refine and evaluate feedback using metrics like factuality, fidelity, helpfulness, and actionability, ultimately aiming to improve feedback quality and timeliness.
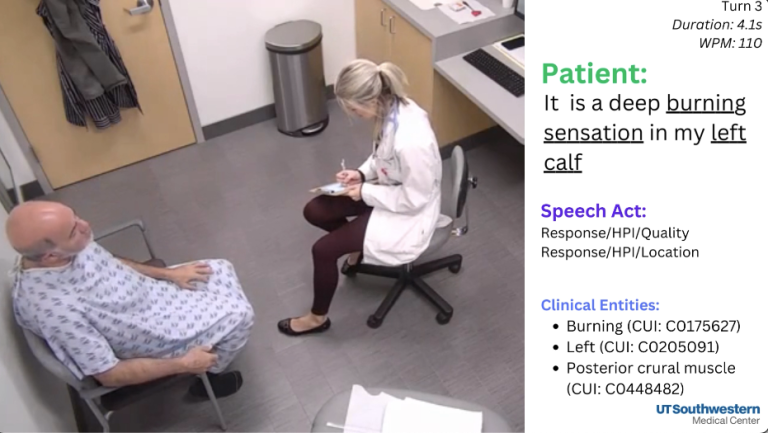
We design machine learning algorithms that annotate and encode clinician-patient interactions so that accurate simulations of various scenarios can be produced for medical student education, practice, and testing.
The video here shows an example of our software annotating an interaction.
This video demonstrates our software annotating a doctor examining a patient via a mesh. You can also watch it on YouTube at https://youtu.be/QV6MFRkN5fc
Prior Projects
The Jamieson Lab also specializes in designing machine learning algorithms and software tools for the following purposes:
- Representing complex spatiotemporal phenomena
- Spatial Biology (2D/3D, multimodal, & highly multiplexed tissue images)
- Advanced Image Analysis (CODEX, GeoMX, Hyperion, and more...)
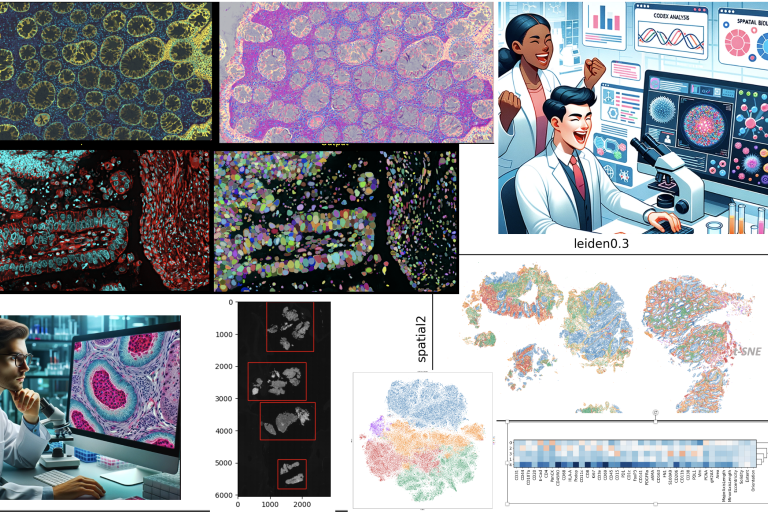
Here is the video abstract for our study Interpretable deep learning uncovers cellular properties in label-free live cell images that are predictive of highly metastatic melanoma.
You can also watch it on YouTube at https://youtu.be/5r-w93dS3Yc
We used a generative deep network to encode latent representation of live-imaged melanoma cells. Supervised machine-learning algorithms classified metastatic efficiency using latent cell representations. We validated classifier prediction on melanoma cell lines in mouse xenografts and interpreted metastasis-driving features in amplified generative cell image models.
Our study also made the cover of the issue.