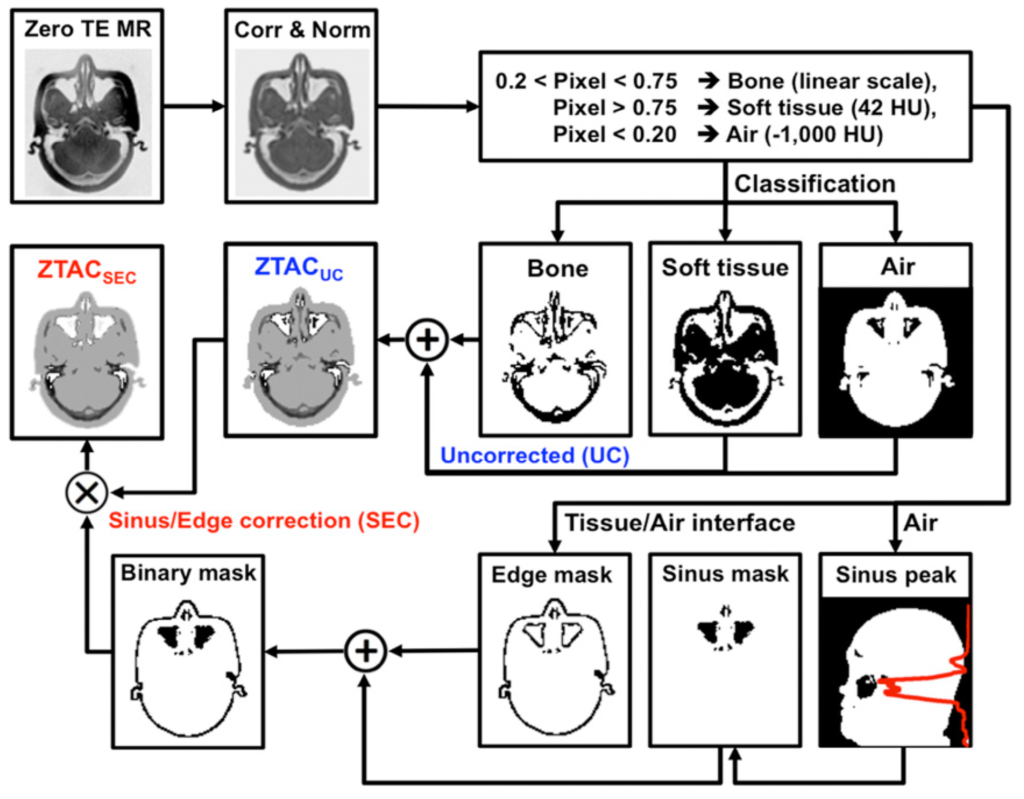
MR-based Attenuation Correction
CT-based attenuation correction (CTAC) is the reference standard for attenuation correction in PET. However, CT is not available in PET/MR systems, and conventional MRI does not provide electron density but proton density. Thus, it is critical to develop an algorithm that can add ”bone signals” into attenuation maps (𝜇-maps) derived from MR images. Zero-echo-time (ZTE)–based attenuation correction (ZTAC) enables the inclusion of bone signals into 𝜇-maps. However, in brain PET/MRI, the major challenge of ZTAC is the misclassification of air/tissue/bone mixtures or their boundaries. Our study evaluated a sinus/edge-corrected (SEC) ZTAC (ZTACSEC), relative to an uncorrected (UC) ZTAC (ZTACUC) and a CT atlas-based attenuation correction (ATAC). ZTACSEC can provide an accurate PET quantification in brain PET/MRI, comparable to the accuracy achieved by CTAC, particularly in the cerebellum.
Direct Attenuation Correction Using Deep Learning
A dedicated stand-alone PET scanner does not come with a combined CT or transmission source, there is no direct solution for accurate attenuation and scatter correction, both of which are critical for quantitative PET. Even in PET/CT, a substantial number of CT examinations are still performed exclusively for PET attenuation correction, a process generally referred to as CTAC, and these scans are redundant if diagnostic CT is performed after PET and/or if diagnostic CT images yield better anatomic information. Thus, if CT- less ASC is feasible at PET/CT, the patient can be spared the additional radiation exposure from CT. Accordingly, removing CTAC has the potential to benefit certain subgroups of patients who may require repetitive PET/CT examinations, especially during childhood, in terms of their long-term health. To address this problem, we proposed joint attenuation and scatter correction (ASC) in the image space for non-corrected PET (PETNC) using deep convolutional neural networks (DCNNs). This approach is a one-step process distinct from conventional methods, done in the sinogram space, that rely on generating attenuation maps first that are then applied to time-consuming iterative scatter simulation. Additionally, our DCNN-based method provides a possible alternative to MRAC.
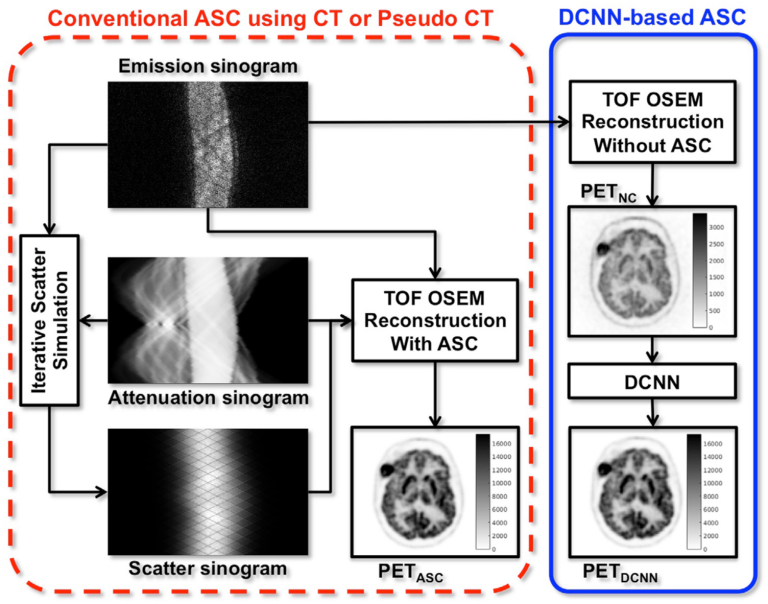
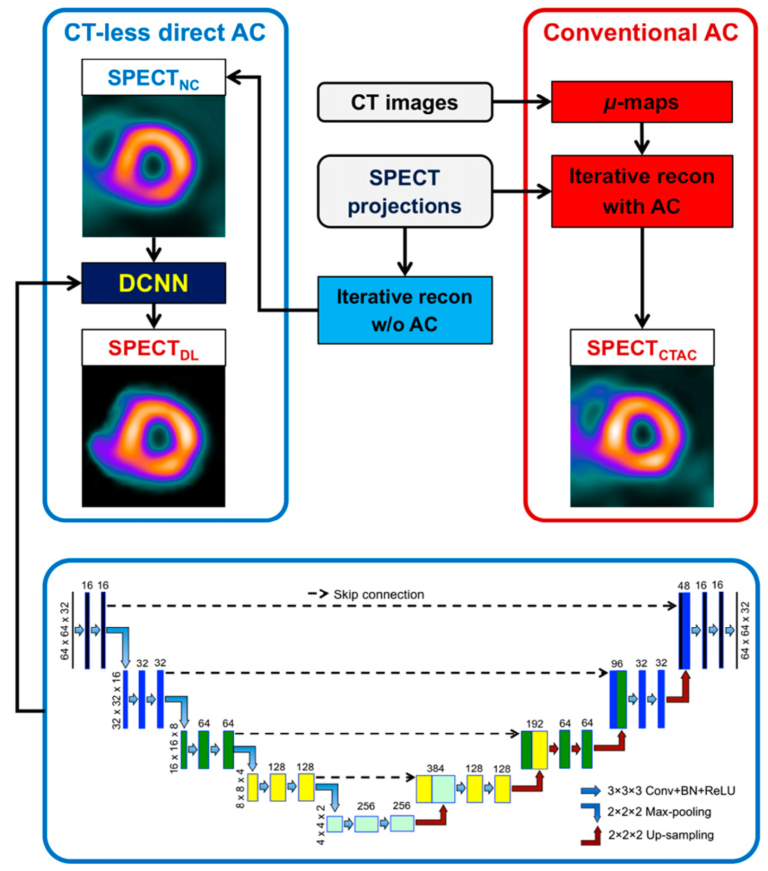
Direct SPECT AC Using Deep Learning
Dedicated cardiac SPECT scanners with cadmium-zinc-telluride cameras have shown capabilities for shortened scan times or reduced radiation doses, as well as improved image quality. Since most dedicated scanners do not have integrated CT, image quantification with attenuation correction (AC) is challenging and artifacts are routinely encountered in daily clinical practice. In this work, we demonstrated a direct AC technique using deep learning (DL) for myocardial perfusion imaging (MPI). Overall, our DL approach reduced attenuation artifacts substantially compared with non-corrected SPECTNC, justifying further studies to establish safety and consistency for clinical applications in stand-alone SPECT systems suffering from attenuation artifacts.