Style Transfer–assisted Deep Learning Method for Kidney Segmentation at Multiphase MRI
We developed and validated a semisupervised style transfer-assisted deep learning method for automated segmentation of the kidneys using multiphase contrast-enhanced (MCE) MRI acquisitions. A cycle-consistent generative adversarial network (CycleGAN) successfully generated anatomically coregistered synthetic multiphase contrast-enhanced (MCE) MRI datasets from T2-weighted MRI acquisitions simulating the precontrast, corticomedullary, early nephrographic, and nephrographic acquisitions. A deep learning segmentation model (mask region–based convolutional neural network [Mask R-CNN]) trained on a synthetic MCE MRI dataset achieved mean Dice scores between 0.91 and 0.93 for kidney segmentation on the original MCE MRI acquisitions.
Guo, et al. Radiol Artif Intell 2023
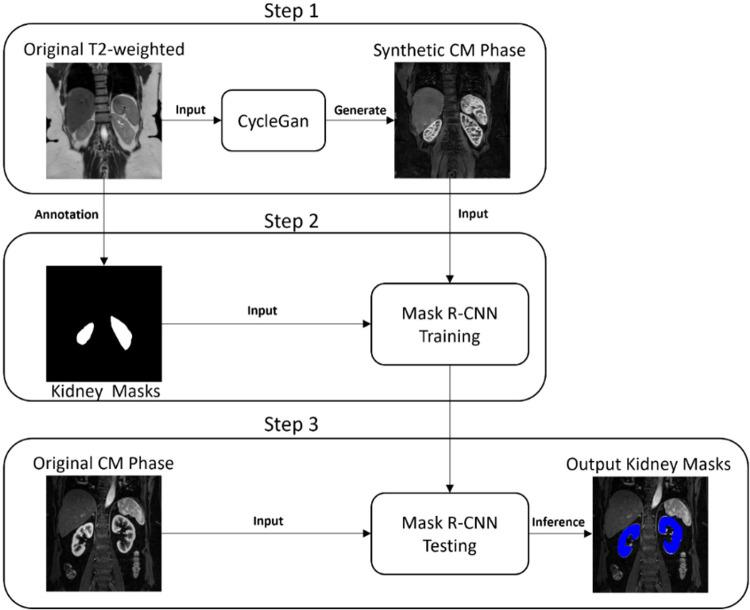
Deep Learning Kidney Segmentation with Very Limited Training Data Using a Cascaded Convolution Neural Network
This study investigated the feasibility of kidney segmentation using deep learning convolution neural network (CNN) models trained with MR images from only a few subjects. Our few-shot kidney segmentation approach using 3D augmentation achieved a good performance even using a single Unet. Furthermore, a cascaded network significantly improved the performance of segmentation and was superior to a single Unet in certain cases.
Guo, et al. PLoS One 2022
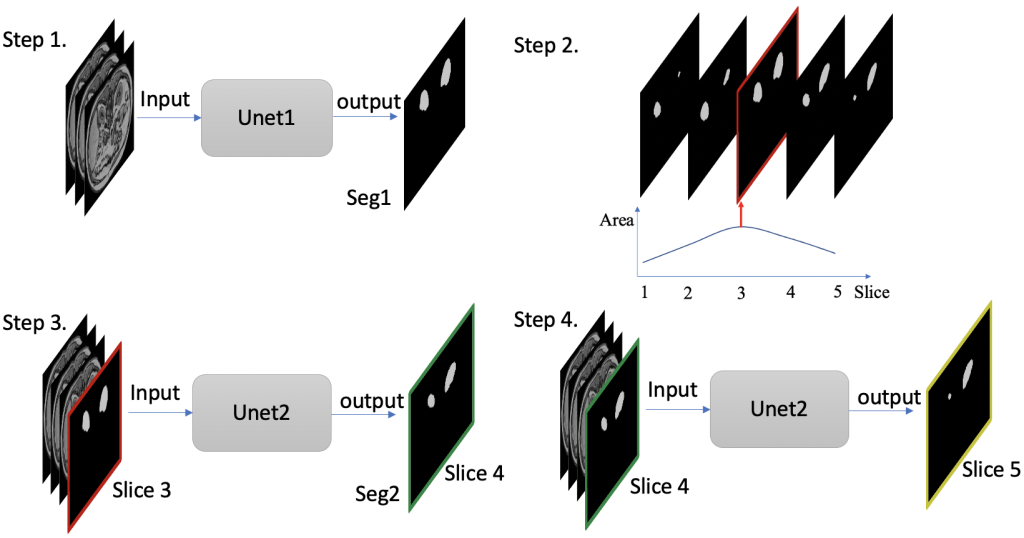
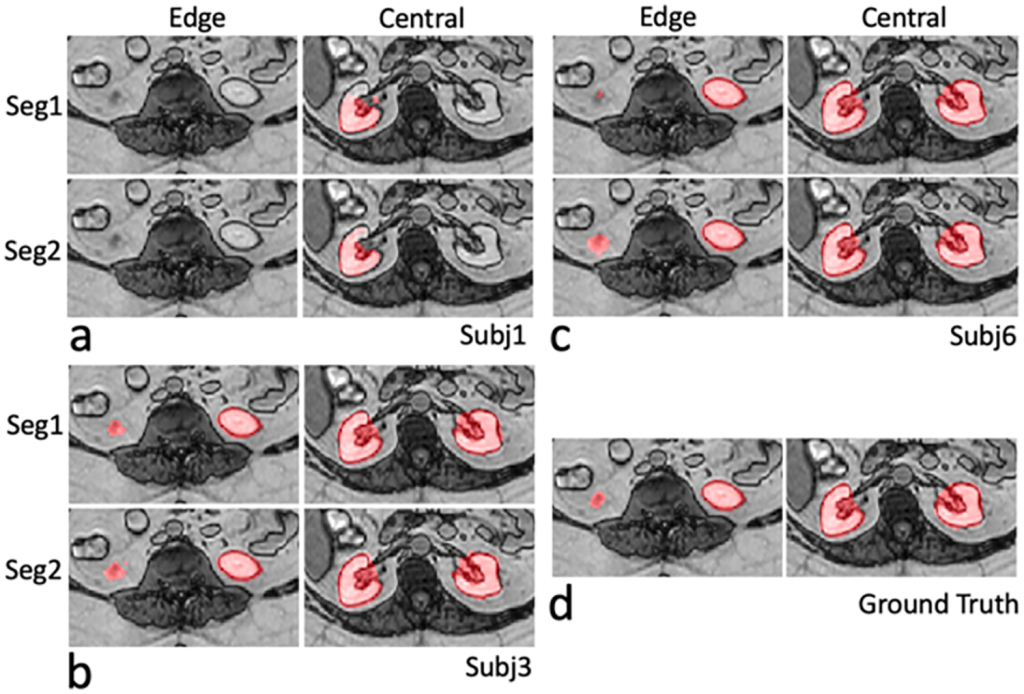
Artificial Intelligence in Kidney Cancer
We review the performance of AI solutions in kidney cancer imaging, with special emphasis on their ability to address some of the unmet clinical needs in the treatment of patients with renal masses, locally advanced kidney cancer, and metastatic disease. We review existing challenges for clinical implementation AI in the radiologic characterization of kidney cancer.
Rasmussen, et al. Am Soc Clin Oncol Educ Book 2022
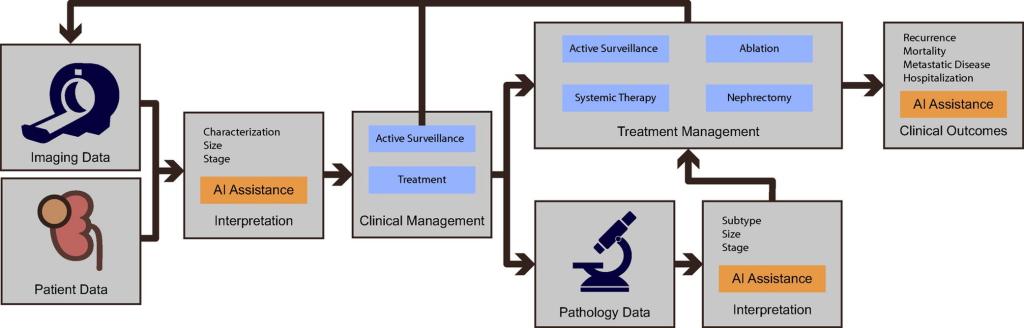
Deep Learning-based Deformable Registration of Dynamic Contrast-Enhanced MR Images of the Kidney
Deformable registration of three-dimensional (3D) dynamic contrast-enhanced (DCE) MRI data improves estimation of kidney kinetic parameters. In this study, we proposed a deep learning approach with two steps: a convolutional neural network (CNN) based affine registration network, followed by a U-Net trained for deformable registration between two MRI images. The proposed registration method was applied successively across consecutive dynamic phases of the 3D DCE-MRI dataset to reduce motion effects in the different kidney compartments (i.e., cortex, medulla).
Huang, et al. Proc SPIE Int Soc Opt Eng 2022
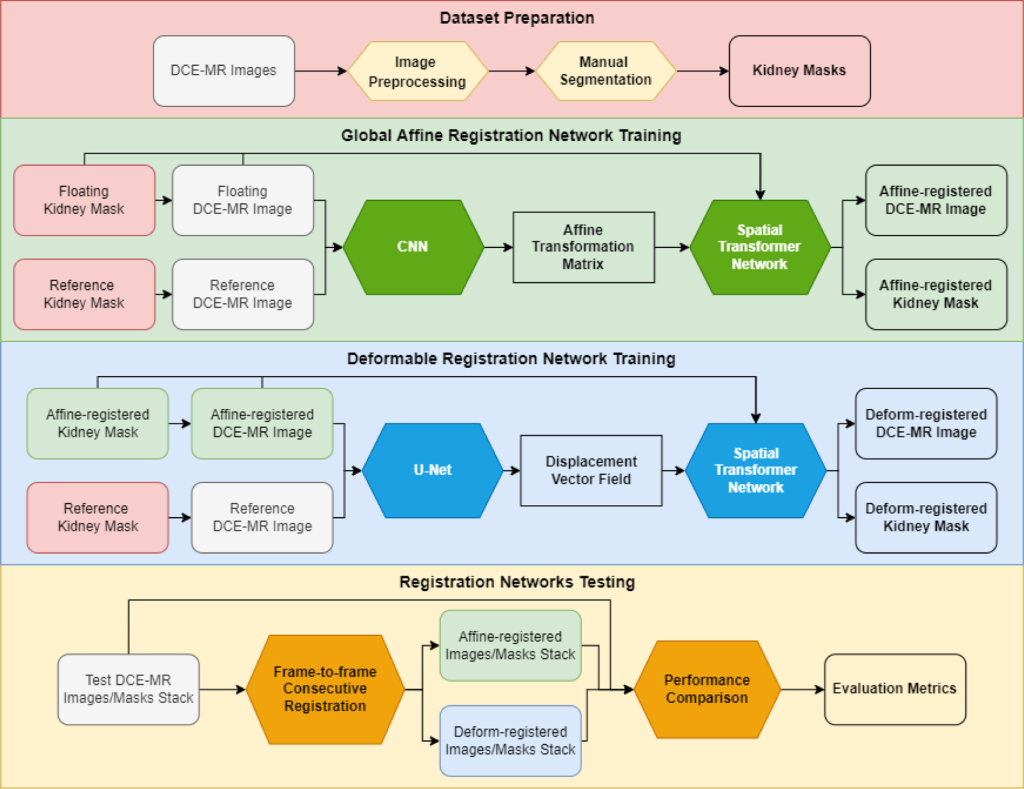
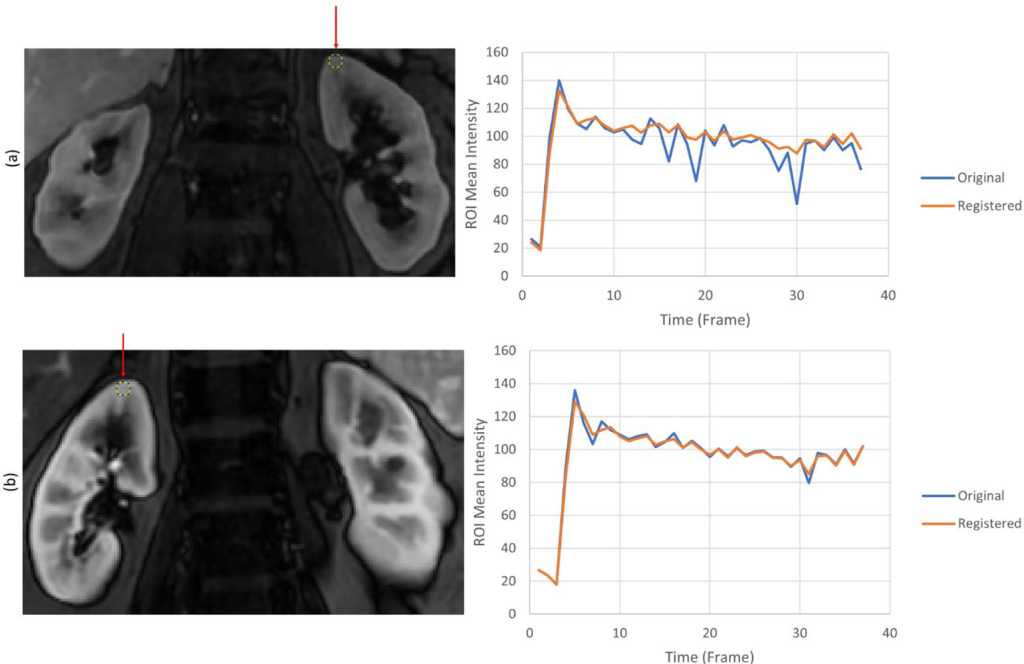